Documents, to Lucene, are the findable items. Here’s where domain-specific abstractions really matter. A Lucene Document can represent a file on a file system, a row in a database, a news article, a book, a poem, an historical artifact (see collections. si.edu), and so on. Documents contain “fields”. Fields represent attributes of the containing document, such as title, author, keywords, filename, file_type, lastModified, and fileSize.
Fields have a name and one or more values. A field name, to Lucene, is arbitrary, whatever you want.
When indexing documents, the developer has the choice of what fields to add to the Document instance, their names, and how they are each handled. Field values can be stored and/or indexed. A large part of the magic of Lucene is in how field values are analyzed and how a field’s terms are represented and structured.
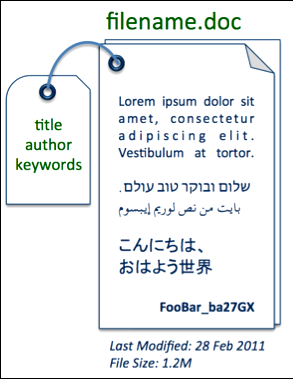
“document” example

There are additional bits of metadata that can be indexed along with the terms text. Terms can optionally carry along their positions (relative position of term to previous term within the field), offsets (character offsets of the term in the original field), and payloads (arbitrary bytes associated with a term which can influence matching and scoring). Additionally, fields can store term vectors (an intra-field term/frequency data structure).
The heart of Lucene’s search capabilities is in the elegance of the index structure, a form of an “inverted index”. An inverted index is a data structure mapping “terms” to the documents. Indexed fields can be “analyzed”, a process of tokenizing and filtering text into individual searchable terms. Often these terms from the analysis process are simply the individual words from the text. The analysis process of general text typically also includes normalization processes (lowercasing, stemming, other cleansing). There are many interesting and sophisticated ways indexing analysis tuning techniques can facilitate typical search application needs for sorting, faceting, spell checking, autosuggest, highlighting, and more.
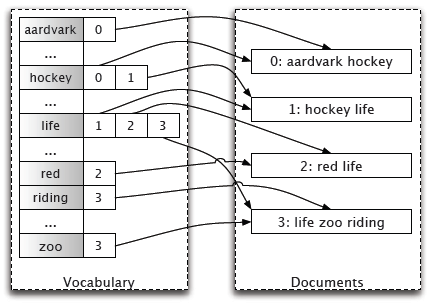
Inverted Index
Again we need to look back at the search application needs. Almost every search application ends up with a human user interface with the infamous and ubiquitous “search box”.

The trick is going from a human entered “query” to returning matching documents blazingly fast. This is where the inverted index structure comes into play. For example, a user searching for “mountain” can be readily accommodated by looking up the term in the inverted index and matching associated documents.
Not only are documents matched to a query, but they are also scored. For a given search request, a subset of the matching documents are returned to the user. We can easily provide sorting options for the results, though presenting results in “relevancy” order is more often the desired sort criteria. Relevancy refers to a numeric “score” based on the relationship between the query and the matching document. (“Show me the documents best matching my query first, please”).
The following formula (straight from Lucene’s Similarity class javadoc) illustrates the basic factors used to score a document.

Lucene practical scoring formula
Each of the factors in this equation are explained further in the following table:
Factor |
Explanation |
score(q,d) |
The final computed value of numerous factors and weights, numerically representing the relationship between the query and a given document. |
coord(q,d) |
A search-time score factor based on how many of the query terms are found in the specified document. Typically, a document that contains more of the query’s terms will receive a higher score than another document with fewer query terms. |
queryNorm(q) |
A normalizing factor used to make scores between queries comparable. This factor does not affect document ranking (since all ranked documents are multiplied by the same factor), but rather just attempts to make scores from different queries (or even different indexes) comparable. |
tf(t in d) |
Correlates to the term’s frequency, defined as the number of times term t appears in the currently scored document d. Documents that have more occurrences of a given term receive a higher score. Note that tf(t in q) is assumed to be 1 and, therefore, does not appear in this equation. However, if a query contains twice the same term, there will be two term-queries with that same term. Hence, the computation would still be correct (although not very efficient). |
idf(t) |
Stands for Inverse Document Frequency. This value correlates to the inverse of docFreq (the number of documents in which the term t appears). This means rarer terms give higher contribution to the total score. idf(t) appears for t in both the query and the document, hence it is squared in the equation. |
t.getBoost() |
A search-time boost of term t in the query q as specified in the query text (see query syntax), or as set by application calls to setBoost(). |
norm(t,d) |
Encapsulates a few (indexing time) boost and length factors. |
Understanding how these factors work can help you control exactly how to get the most effective search results from your search application. It's worth noting that in many applications these days, there are numerous other factors involved in scoring a document. Consider boosting documents by recency (latest news articles bubble up), popularity/ratings (or even like/dislike factors), inbound link count, user search/click activity feedback, profit margin, geographic distance, editorial decisions, or many other factors. But let's not get carried away just yet, and focus on Lucene's basic tf/idf.
So now we've briefly covered the gory details of how Lucene works for matching and scoring documents during a search. There's one missing bit of magic, going from the human input of a search box and translating that into a representative data structure, the Lucene Query object. This string, Query process is called "query parsing". Lucene itself includes a basic QueryParser that can parse sophisticated expressions including AND, OR, +/-, parenthetical grouped expressions, range, fuzzy, wild carded, and phrase query clauses. For example, the following expression will match documents with a title field with the terms "Understanding" and Lucene collocated successively (provided positional information was enabled!) where the mimeType (MIME type is the document type) value is "application/pdf":
title:”Understanding Lucene” AND mimeType:application/PDF
For more information on Lucene QueryParser syntax, see http://lucene.apache.org/java/3_0_3/queryparsersyntax.html (or the docs for the version of Lucene you are using).
It is important to note that query parsing and allowable user syntax is often an area of customization consideration. Lucene’s API richly exposes many Query subclasses, making it very straightforward to construct sophisticated Query objects using building blocks such as TermQuery, BooleanQuery, PhraseQuery, WildcardQuery, and so on.
Shining the Light on Lucene: Solr
Apache Solr embeds Java Lucene, exposing its capabilities through an easy-to-use HTTP interface. Solr has Lucene best practices built in, and provides distributed and replicated search for large scale power.
For the examples that follow, we’ll be using Solr as the front-end to Lucene. This allows us to demonstrate the capabilities with simple HTTP commands and scripts, rather than coding in Java directly. Additionally, Solr adds easy-to-use faceting, clustering, spell checking, autosuggest, rich document indexing, and much more. We’ll introduce some of Solr’s value-added pieces along the way.
Lucene has a lot of flexibility, likely much more than you will need or use. Solr layers some general common-sense best practices on top of Lucene with a schema. A Solr schema is conceptually the same as a relational database schema. It is a way to map fields/ columns to data types, constraints, and representations. Let’s take a preview look at fields defined in the Solr schema (conf/schema. xml) for our running example:
<fields>
<field name=”id”
type=”string” indexed=”true” stored=”true”/>
<field name=”title”
type=”text_en” indexed=”true” stored=”true” />
<field name=”mimeType”
type=”string” indexed=”true” stored=”true” />
<field name=”lastModified”
type=”date” indexed=”true” stored=”true” />
</fields>
The schema constrains all fields of a particular name (there is dynamic wildcard matching capability too) to a “field type”. A field type controls how the Lucene Field instances are constructed during indexing, in a consistent manner. We saw above that Lucene fields have a number of additional attributes and controls, including whether the field value is stored, indexed, if indexed, how so, which analysis chain, and whether positions, offsets, and/or term vectors are stored.
Our Running Example, Quick Proof-of-Concepts
The (Solr) documents we index will have a unique “id” field, a “title” field, a “mimeType” field to represent the file type for filtering/faceting purposes, and a “lastModified” date field to represent a file’s last modified timestamp. Here’s an example document (in Solr XML format, suitable for direct POSTing):
<add>
<doc>
<field name=”id”>doc01</field>
<field name=”title”>Our first document</field>
<field name=”mimeType”>application/pdf</field>
<field name=”lastModified”>NOW</field>
</doc>
</add>
That example shows indexing the metadata regarding an actual file. Ultimately, we also want the contents of the file to be searchable. Solr natively supports extracting and indexing content from rich documents. And LucidWorks Enterprise has built-in file and web crawling and scheduling along with content extraction.
Launching Solr, using its example configuration, is as straightforward as this, from a Solr installation directory:
cd example
java –jar start.jar
And from another command-shell, documents can be easily indexed. Our example document shown previously (saved as docs.xml for us) can be indexed like this:
cd example/exampledocs
java –jar post.jar docs.xml
First of all, this isn’t going to work out of the box, as we have a custom schema and applications needs not supported by Solr’s example configuration. Get used to it, it’s the real world! The example schema is there as an example, and likely inappropriate for your application as-is. Borrow what makes sense for your own applications needs, but don’t leave cruft behind.
At this point, we have a fully functional search engine, with a single document, and will use this for all further examples. Solr will be running at http://localhost:8983/solr.
{{ parent.title || parent.header.title}}
{{ parent.tldr }}
{{ parent.linkDescription }}
{{ parent.urlSource.name }}